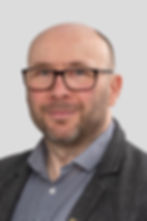
Reading tissue samples for breast cancer can be subjective and therein lies a problem – lack of standardisation.
Oestrogen receptor positive Her2 negative is the most common breast cancer in New Zealand and globally but specialists don’t want to prescribe chemotherapy unnecessarily because it can be highly toxic. Neither do they want to miss someone who might benefit. If this type of cancer recurs, 50 per cent return after five years.
This scenario sits within a much wider picture – increasing rates of cancer, including breast cancer, and the consequent rise in biopsies, interventions and treatment. We have a global shortage of pathologists. Clinicians want more and more data. Our public health system laboratories need digital platforms. And we need to do all this and keep the research going.
Pathologist Gavin Harris from Canterbury Health Laboratories at Christchurch Public Hospital says these are the parameters shaping the future, a ‘perfect storm of increasing need for accuracy against reducing resource’. But he may have a solution using AI and machine learning in an emerging discipline called computational pathology.
Harris has worked in pathology since graduating in the UK. He emigrated to New Zealand in late 2003 and has been the lead breast cancer pathologist at Canterbury Health Laboratories since then. He led the development of the specialist breast pathology service and was the director of anatomical pathology for six years. He has been involved in multiple national working groups on breast cancer pathology and was the lead in establishing the Christchurch Breast Cancer register, now part of the Breast Cancer Foundation National Register. He has received five Health Research Council grants for research related to computational pathology/computer-assisted diagnosis and breast cancer, working with the universities of Canterbury and Auckland. He is also currently working with Te Aho o Te Kahu – Cancer Control Agency as an advisor.
The current system involves laboratory testing which is laborious, time-consuming and has poor reproducibility, or the patient can pay more than US$3,100 and wait two weeks for a test done in the US, raising issues of equity.
“Computational pathology offers ways to quantify the changes we see under the microscope with an unprecedented level of accuracy using algorithms to enhance the information pathologists can glean from cancer samples,” says Harris. “Better accuracy means we’re less likely to miss someone because of a subjective opinion.
“We’re also going to save time and resources using these approaches. It can take up to two hours to manually review a tissue sample and produce a report for clinicians. Computational pathology could reduce that time, freeing up scarce specialist resources.
"More tailored information more quickly means a more accurate diagnosis, improved prediction of outcome and response to treatments, and optimised patient outcomes.”
He says there’s been a lot of literature that suggests that by using the quantitative power of AI and machine learning we can get biological signatures and surrogate biological data from our basic stain which is very useful for oncologists in the breast cancer setting. “With oestrogen receptor positive HER2 negative, we’re trying to identify the wolf in sheep’s clothing, an aggressive subtype called luminal B which indicates an increased likelihood of recurrence.”
Harris has Research Accelerator Programme (RAP) stage II funding for this research. He has already trialled computational pathology on a trial set of 59 cases, gaining over 85 per cent accuracy. The next step is to train the algorithm on a purely New Zealand cohort using tissue samples from the He Taonga Tapu Cancer Society Christchurch Tissue Bank.
Harris says they’re also applying to KiwiNet for additional funding to prepare for investor activity in 2025 when he hopes to spin out the company. He expects to file a patent application in the not-too-distant future; currently, the FDA has approved one such algorithm for prostate cancer.
Harris admits that commercialisation is something quite foreign to him, and that he’s been extremely lucky throughout this process with support from groups such as Via Innovations and the Health Innovation Hub. “I turned up at Via there saying I had this great idea. Associate Professor Helen Lunt from Health New Zealand/University of Otago has provided much needed mentoring and put me in touch with Matt Nicholson at the Hub.”
“He organised some Tier 1 funding from KiwiNet and now we’re looking at Tier 2 funding. He’s gone above and beyond the call of duty to help with the commercialisation journey – what it looks like, what we should be doing, how we should be thinking about it.”
He also credits Health New Zealand as being outstanding along with the MedTech team, Professor Ramakrishn Mukundan and Andrew Davidson in the Dept of Computer Science and Software Engineering at the University of Canterbury. Professor Cris Print and team at the University of Auckland also helped with genomic testing and analysis.